Hey Hi! AI: Can't Reason, So No Fears!
- Borrow2Share
- Nov 21, 2023
- 3 min read
Updated: Oct 6, 2024
11.2023. Hey Hi! AI. Still Much Ado About Nothing For Now. It Can't Reason, So No Fears! Forget All The (A)rtificial (I)Flation and Sci-Fi Fantasies.
ChatGPT Overview. A Great Assistant & Helper. Imitation Learning w/ User-Expert Dialogues and Sample Problems,Solutions,Steps. And Reinforced Learning w/ Human Feedback Automated.
Yet, There Is Still No True Reasoning Abilities Behind The Machine! But Voluminous Memorization, Hyper Patterns Matching, Tremendous Finding Relationships Between Words, Etc.
LLMs Can Be Trained For Human Preferences/Values and Data Truthfulness/Helpfulness/Harmlessness. Maybe Love & Grace In The Machine.
A Skills Match Between an LLM and You. Will Probably Beat You at Scramble Word Games & Chess Matches. But, Still No Match For Your Reasonings and Creativity by Decades.
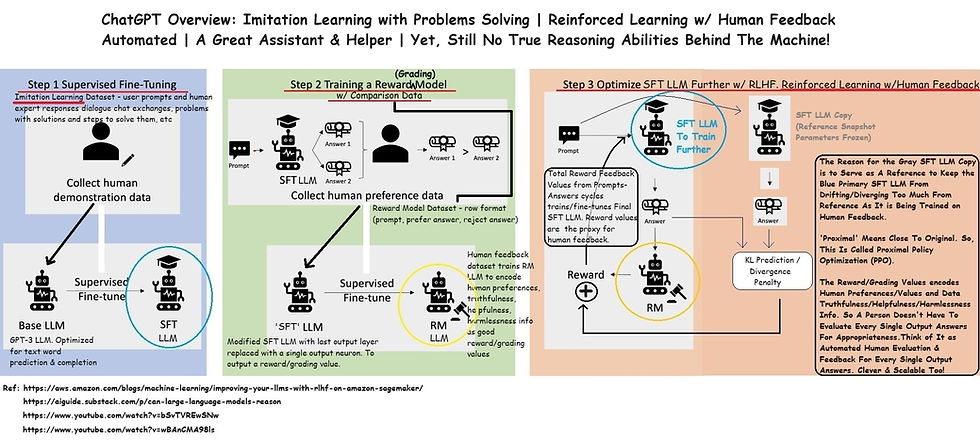
Improving your LLMs with RLHF on Amazon SageMaker
Can Large Language Models Reason?
How ChatGPT Works Technically | ChatGPT Architecture.
How To Prompt ChatGPT Properly & Perfectly.
What Are LLM Parameters and Tokens? Both Weights & Biases Characterizing Each And Every Neuron In the LLM Neural Network.
Yes, the parameters in a large language model (LLM) are similar to the weights in a standard neural network. In both LLMs and neural networks, these parameters are numerical values that start as random coefficients and are adjusted during training to minimize loss. These parameters include not only the weights that determine the strength of connections between neurons but also the biases, which affect the output of neurons. In a large language model (LLM) like GPT-4 or other transformer-based models, the term "parameters" refers to the numerical values that determine the behavior of the model. These parameters include weights and biases, which together define the connections and activations of neurons within the model. Here's a more detailed explanation:
Weights: Weights are numerical values that define the strength of connections between neurons across different layers in the model. In the context of LLMs, weights are primarily used in the attention mechanism and the feedforward neural networks that make up the model's architecture. They are adjusted during the training process to optimize the model's ability to generate relevant and coherent text.
Biases: Biases are additional numerical values that are added to the weighted sum of inputs before being passed through an activation function. They help to control the output of neurons and provide flexibility in the model's learning process. Biases can be thought of as a way to shift the activation function to the left or right, allowing the model to learn more complex patterns and relationships in the input data.
The training process involves adjusting these parameters (weights and biases) iteratively to minimize the loss function. This is typically done using gradient descent or a variant thereof, such as stochastic gradient descent or Adam optimizer. The loss function measures the difference between the model's predictions and the true values (e.g., the correct next word in a sentence). By minimizing the loss, the model learns to generate text that closely resembles the patterns in its training data.
Researchers often use the term "parameters" instead of "weights" to emphasize that both weights and biases play a crucial role in the model's learning process. Additionally, using "parameters" as a more general term helps communicate that the model is learning a complex set of relationships across various elements within the architecture, such as layers, neurons, connections, and biases.
Three Different Types Of LLMs. Google PaLM API and MakerSuite To Create Gen AI Apps. Tasks Tuned For. Input Prompts. Instruction, Context, and Data.
From Traditional Programming -->> Neural Networks. Object Recognition/Detection -- >> Generative ML/AI. Creating New Content!
How Are LLMs Stored, Loaded, And Executed?
GGML. GGUF. Paramter File. Runtime File.
ATechnical User's Introduction to Large Language Models (LLMs)
LLMs: Data Privacy and Security!
Once Data Is Fed Into The LLM, You Can't Get It Out. No Delete Button.
Can't Separate The Data From The Model. So Sanitize Training Data First!
Comments